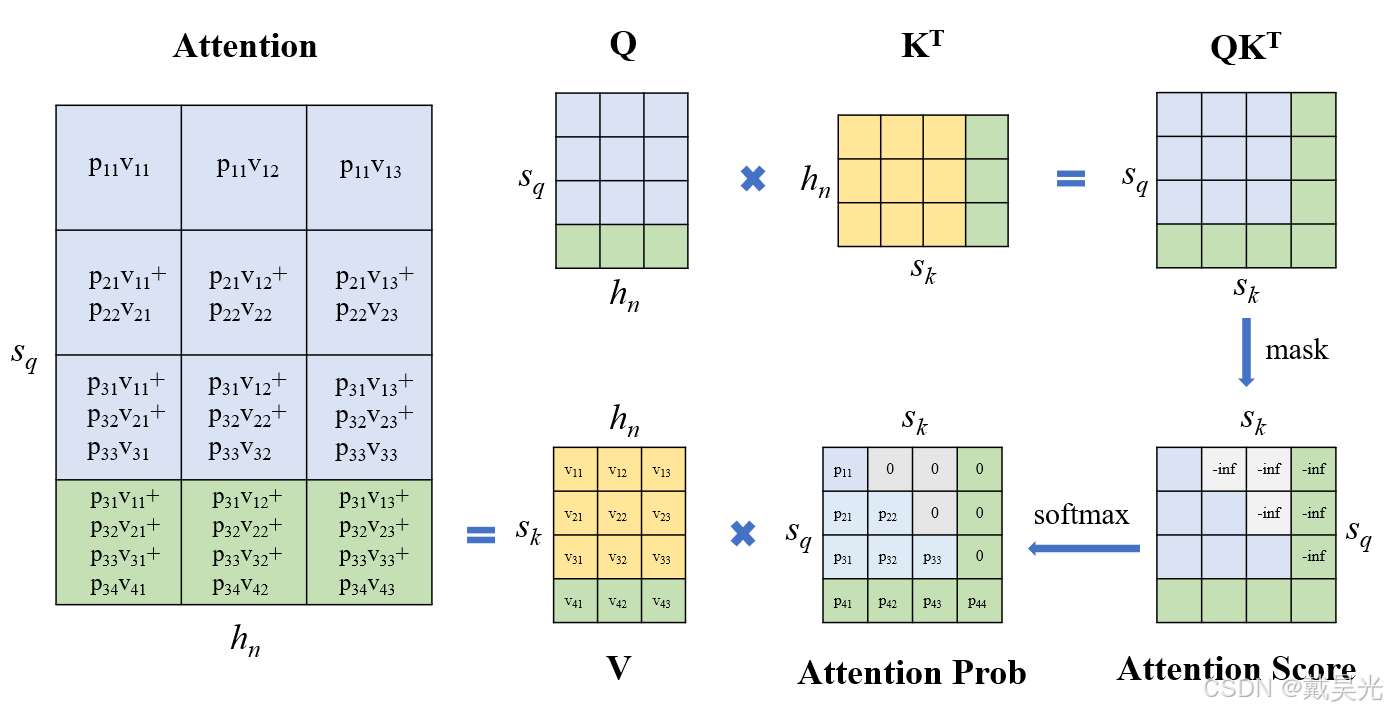
GLM-4 (6) - KV Cache / Prefill & Decode
KV Cache是一种为大模型量身定制的推理加速技术。为什么?因为大模型推理的逻辑是:根据当前轮输入tokens预测并输出下一个token,这两者拼接就得到了下一轮的输入,每一轮只比上一轮增加了一个token。这意味着当前轮包含了上一轮的部分计算。上一轮中每一层的key和value被当前轮复用,而不是重新计算,就能加速推理过程,这就是KV Cache的作用。随着KV Cache的广泛使用,大模型的
系列文章目录
GLM-4 (1) - 推理+概览
GLM-4 (2) - RoPE
GLM-4 (3) - GLMBlock
GLM-4 (4) - SelfAttention
GLM-4 (5) - API & Function Calling
GLM-4 (6) - KV Cache / Prefill & Decode
前言
KV Cache
是一种为大模型量身定制的推理加速技术。为什么?因为大模型推理的逻辑是:根据当前轮输入tokens
预测并输出下一个token
,这两者拼接就得到了下一轮的输入,每一轮只比上一轮增加了一个token
。这意味着当前轮包含了上一轮的部分计算。上一轮中每一层attention layer
的key
和value
被当前轮复用,而不是重新计算,就能加速推理过程,这就是KV Cache
的作用。
随着KV Cache
的广泛使用,大模型的推理被分为了Prefill
和Decode
两个阶段。简单来说,Prefill
阶段将prompt
喂给模型做forward计算,该过程一次性计算了每个prompt token
对应的key
和value
,并且被缓存起来;在Decode
阶段,模型每foward
一次生成一个token
。这两个阶段有较为明显的差异。
本文的内容主要包含以下两方面:1)笔者曾对KV Cache
的可行性
有所怀疑(当然这是因为当时没想明白,后面会讲到),我会将阐述我当时的困惑,并结合代码分析证明KV Cache
的可行性;2)针对Prefill
和Decode
的不同特点,目前已经有一些serve
上的优化,因此想更详细的说一下这两个阶段。
一、KV Cache
1.可行性疑问
KV cache
的可行性值得推敲的地方如下。大模型通常有N
个(Transformer) Block
级联,每个Block
中都有一个attention layer
。对于第一个Block
,缓存key
和value
容易理解;但是从第二个Block
开始,它们的输入都是上一个Block
的输出,只要第一个Block
输出发生变化,后面的Block
中的key
和value
就发生变化,此时KV Cache
就无法成立。乍一想,计算attention score
的时候多一个token
,softmax
计算不得变化嘛!值得一提的是,在这篇KV Cache的文章下面有一条来自用户"QQ用户889083339"评论,也提及了同样的困惑。
主要的问题在于,乍一想属于想当然,说明对decode-only
架构的大模型的masked attention
的计算还不够熟悉。下面我们来分析一下,证明KV Cache
是可行的。
2.可行性分析
假设当前轮forward
时序列长度是s
,那么根据GLM-4
的代码并沿用其中对变量的符号表示,可知计算得到的Attention
矩阵的形状是[b, np, sq, hn]
。其中b
是batch_size
,np
是多头注意力头的个数,sq = sk = s
就是当前序列长度,只是针对key
和query
分别使用下标k
和q
来表示,hn
是单个注意力头对应的维度。
为方便后面分析,我们刨除前面的b
和np
这两个维度,则当前轮Attention
的形状为[sq, hn]
,如下图中左侧的Attention部分所示;那么下一轮序列长度增加1,形状变成了[s+1, hn] = [sq', hn]
。图中Attention
蓝色部分表示当前轮需要计算的部分,而绿色部分则表示下一轮新添加的部分。
想要证明KV cache
是可行的,就是要证明当前轮和下一轮在Attention
的蓝色部分计算结果是一致的。因为这部分一致保证了前后两轮在级联的Block
中attention layer
中key
和value
的前sk
个也是一致的。接下来我们就跟随Attention
的计算过程来验证这件事情。计算过程如上图所示,对应GLM-4
中CoreAttention
的计算过程(代码贴在下方):
- 计算 Q K T QK^{T} QKT:
[sq, hn] * [hn, sk] --> [sq, sk]
; - 对 Q K T QK^{T} QKT进行
mask
操作,得到注意力分数Attention Score
:[sq, sk] --> [sq, sk]
,因为decode-only
做的是单向掩码,将上三角部分全部置成负无穷; - 使用
Softmax
计算概率Attention Prob
:[sq, sk] --> [sq, sk]
,此时上三角的概率都为0; - 计算
Attention
:Attention Prob * V
,[sq, sk] * [sk, hn] --> [sq, hn]
。
我们发现KV cache
之所以可行,与mask
操作有关:
1)不管是当前轮还是下一轮,Attention Prob
矩阵的第一行,都只会有一个非零元素p11
,它的值始终都是1,因为是单项的,能看到key
只有一个;同样的,对于第二行(对应第二个query
),只能看到前面的两个key
,以此类推;如果你还没有明白,那么请验证从在执行Softmax
操作时,Attention Score
添加绿色部分(新一轮),不会改变Attention Prob
蓝色部分的结果;
2)在计算Attention
的时候,同样可以验证新一轮计算其蓝色部分结果不变,因为只能看到前面的value
。
至此,我们已经证明了KV Cache
是可行的。总结一下就是,mask
操作是单向的,这使得Attention
计算只会在sq
方向上不断拼接,而不改变前面的计算结果。
# CoreAttention
class CoreAttention(torch.nn.Module):
def __init__(self, config: ChatGLMConfig, layer_number):
super(CoreAttention, self).__init__()
self.config = config
self.apply_query_key_layer_scaling = config.apply_query_key_layer_scaling # True
self.attention_softmax_in_fp32 = config.attention_softmax_in_fp32 # True
if self.apply_query_key_layer_scaling:
self.attention_softmax_in_fp32 = True
self.layer_number = max(1, layer_number) # GLMBlock或者是attention的层数(1~40)
self.is_causal = True
projection_size = config.kv_channels * config.num_attention_heads # 投影层尺寸:4096 = 128 * 32
# Per attention head and per partition values.
self.hidden_size_per_partition = projection_size # 4096
self.hidden_size_per_attention_head = projection_size // config.num_attention_heads # 根据头的个数均分:128 = 4096 // 32
self.num_attention_heads_per_partition = config.num_attention_heads # 32
coeff = None
self.norm_factor = math.sqrt(self.hidden_size_per_attention_head) # 注意力公式种的根号d
if self.apply_query_key_layer_scaling: # 不同的层,会有不同的缩放系数(很熟悉,但是需要找找出处)
coeff = self.layer_number
self.norm_factor *= coeff # TODO: WHY
self.coeff = coeff
self.attention_dropout = torch.nn.Dropout(config.attention_dropout)
def forward(self, query_layer, key_layer, value_layer, attention_mask):
# [b, np, sq, sk] 即(batch_size, num_head, seq_len, seq) (1, 32, 8, 8)。这边query_layer和key_layer形状是相同的
output_size = (query_layer.size(0), query_layer.size(1), query_layer.size(2), key_layer.size(2))
# [b, np, sq, hn] -> [b * np, sq, hn] (1, 32, 8, 128) -> (32, 8, 128)
query_layer = query_layer.view(output_size[0] * output_size[1], output_size[2], -1)
# [b, np, sk, hn] -> [b * np, sk, hn] (1, 32, 8, 128) -> (32, 8, 128)
key_layer = key_layer.view(output_size[0] * output_size[1], output_size[3], -1)
# preallocting input tensor: [b * np, sq, sk] query_layer和key_layer矩阵相乘 -> (1*32, 8, 8)
matmul_input_buffer = torch.empty(
output_size[0] * output_size[1], output_size[2], output_size[3], dtype=query_layer.dtype,
device=query_layer.device
)
# Raw attention scores. [b * np, sq, sk]
matmul_result = torch.baddbmm(
matmul_input_buffer, # 这个量不需要管,因为与之相乘的beta=0.0
query_layer, # [b * np, sq, hn]
key_layer.transpose(1, 2), # [b * np, hn, sk]
beta=0.0,
alpha=(1.0 / self.norm_factor), # 这边矩阵batch相乘有个缩放系数
)
# change view to [b, np, sq, sk]
attention_scores = matmul_result.view(*output_size)
# ===========================
# Attention probs and dropout
# ===========================
# attention scores and attention mask [b, np, sq, sk]
if self.attention_softmax_in_fp32:
attention_scores = attention_scores.float() # (1, 32, 8, 8)
if self.coeff is not None:
attention_scores = attention_scores * self.coeff
if attention_mask is None and attention_scores.shape[2] == attention_scores.shape[3]:
attention_mask = torch.ones(output_size[0], 1, output_size[2], output_size[3],
device=attention_scores.device, dtype=torch.bool) # (batch_size, 1, seq_len, seq_len)
attention_mask.tril_() # 下三角都为True,其余是False
attention_mask = ~attention_mask # 取反,下三角都是False
if attention_mask is not None:
attention_scores = attention_scores.masked_fill(attention_mask, float("-inf")) # 下三角保持,其余部分注意力分数都为-inf。也就是单向的
attention_probs = F.softmax(attention_scores, dim=-1) # (1, 32, 8, 8)
attention_probs = attention_probs.type_as(value_layer)
# This is actually dropping out entire tokens to attend to, which might
# seem a bit unusual, but is taken from the original Transformer paper.
attention_probs = self.attention_dropout(attention_probs)
# query layer shape: [b * np, sq, hn]
# value layer shape: [b, np, sk, hn]
# attention shape: [b, np, sq, sk]
# context layer shape: [b, np, sq, hn]
output_size = (value_layer.size(0), value_layer.size(1), query_layer.size(1), value_layer.size(3)) # (batch_size, num_head, seq_len, dim_per_head) (1, 32, 8, 128)
# change view [b * np, sk, hn]
value_layer = value_layer.view(output_size[0] * output_size[1], value_layer.size(2), -1) # (1 * 32, 8, 128)
# change view [b * np, sq, sk]
attention_probs = attention_probs.view(output_size[0] * output_size[1], output_size[2], -1) # (1 * 32, 8, 8)
# matmul: [b * np, sq, hn]
context_layer = torch.bmm(attention_probs, value_layer) # (1 * 32, 8, 128)
# change view [b, np, sq, hn]
context_layer = context_layer.view(*output_size) # (1, 32, 8, 128)
# [b, np, sq, hn] --> [b, sq, np, hn]
context_layer = context_layer.transpose(1, 2).contiguous() # 序列维度前置,(1, 8, 32, 128)
# [b, sq, np, hn] --> [b, sq, hp]
new_context_layer_shape = context_layer.size()[:-2] + (self.hidden_size_per_partition,) # 多头合并,(1, 8, 4096)
context_layer = context_layer.reshape(*new_context_layer_shape)
return context_layer
二、Prefill & Decode
1.演示
Prefill
:预填充阶段,把整段prompt
喂给模型并执行一次前向过程,直至生成第一个token
,也就是图中的t3
;这个阶段一次性计算了prompt
中所有的KV Cache
;Decode
:解码阶段,逐个token
输出,如黄色部分所示;这个阶段使用了之前计算好的KV Cache
加速推理,同时当前forward
过程中计算的新的key
和value
也被缓存起来,与之前的KV Cache
拼接起来,以便下一轮使用。
在GLM-4 (1) - 推理+概览
这篇文章的prepare_inputs_for_generation() & kv_cache
小节,我们观察了第一次forward
和第二次forward
时的输入,如下所示。
# 首次forward时返回结果(输入为it is me):
{
"input_ids": tensor([[151331, 151333, 151336, 198, 275, 374, 752, 151337]], device='cuda:0'),
"past_key_values": None,
"position_ids": tensor([[0, 1, 2, 3, 4, 5, 6, 7]], device='cuda:0'),
"attention_mask": tensor([[1, 1, 1, 1, 1, 1, 1, 1]], device='cuda:0'),
"return_last_logit": True,
"use_cache": True
}
# 当生成一个token,第二次forward时返回结果:
{
"input_ids": tensor([[198]], device='cuda:0'), # 新生成的token
"past_key_values": [40 * tuple(2 * torch.Size([1, 2, 8, 128]))],
"position_ids": tensor([[8]], device='cuda:0'),
"attention_mask": tensor([[1, 1, 1, 1, 1, 1, 1, 1, 1]], device='cuda:0'),
"return_last_logit": True,
"use_cache": True
}
- 首次
forward
对应的就是Prefill
阶段,输入的是整个prompt
,长度为8,同时past_key_values = None
,因为还没有KV Cache
; - 第二次forward对应的就是
Decode
阶段,输入只是上一次生成的单个token
,past_key_values
的shape是num_layers * 2 * batch_size * num_groups * seq_len * hn
,其中num_layers
是GLMBlock
个数或者Attention layer
的层数,num_groups
是Grouped-query attention
的组数。
2.性能指标
由于推理存在这两个不同的阶段,因此他们分别会对应不同的性能指标,这些指标也就是当下一些serve
框架在优化的目标。比如之前挺火的Kimi
的Mooncake
,是Prefill
和Decode
分离的架构,就是针对不同阶段的不同特性进行优化,提升性能,当然就包括下面会提及的性能指标(当然这里主要考虑有大量请求的情况)。
Prefill
性能指标:TTFT (Time To First Token)
,表示生成第1个token
所需的时间。Decode
性能指标:TPOT (Time Per Output Token)
,Decode
阶段每生成一个token
所用的时间。
总结
本篇首先给出了之前关于KV Cache
可行性的困惑,并通过Attention
计算图解的方式给出了答案,加深了对KV Cache
的理解;同时展示了LLM
推理的两个阶段Prefill & Decode
,并介绍了它们的性能指标,对大模型推理过程有了更深入的理解。
更多推荐
所有评论(0)